5 Potential solutions
The theoretical results from Section 3 suggest a number of potential methods to avoid, correct, or otherwise mitigate the errors created by taxonomic bias.
5.1 Use ratio-based relative-abundance analyses
Since bias creates a consistent fold error in species ratios, a natural approach to countering bias when analyzing relative abundances is to use DA methods that are based on fold changes in ratios. A variety of such methods have been developed for microbiome DA analysis that build on earlier work from the field of Compositional Data Analysis (CoDA). These methods analyze log-fold changes in the ratios among a pair of species or, more generally, some product of exponential power of multiple species, which likewise are bias-invariant. Besides a greater robustness to taxonomic bias, such ratio-based analyses have other advantages. First, analysis of ratios avoids other standard criticisms leveled at proportion-based analysis—namely that the change in proportion of a species is affected by change in the density of all other species and the set of species used in the denominator of the proportion calculation (Gloor et al. (2017)). Second, ratios may be more informative than absolute density in some cases, such as for swabs or other sample types for which the absolute density in the sample may be an unreliable indicator of the absolute density of the sampled ecosystem.
Ratios come with their limitations, however. First, there are a vast number of species ratios (and derivatives) to potentially consider, none of which provide direct information about absolute densities, which can make choosing and interpreting a ratio-based DA analysis challenging. Second, ratio measurements are impacted by noise in both the numerator and denominator and so can be noisier than proportion measurements, particularly when the read counts in the denominator are small. A related problem is that, due to the discrete nature of biological organisms and the reads that are generated during sequencing, species in the denominator are commonly observed to have 0 reads; the assumptions that made by different methods about the meaning of these 0 counts can have a major impact on DA results. Finally, bias invariance at the level of ratios among species does not extend to ratios of additive aggregates of species, such as between bacterial phyla, unless further conditions are met (McLaren, Willis, and Callahan (2019)).
5.2 Calibration using community controls
Measurement of community calibration controls along with the primary experimental samples can enable researchers to directly measure and remove the effect of bias prior to or concurrent with downstream DA analysis. Community calibration controls are samples whose species identities and relative abundances are known either by construction or by characterization with a chosen ‘gold standard’ or reference protocol (McLaren, Willis, and Callahan (2019)). MGS measurement of one or more control communities can be used to directly measure the relative efficiencies among the superset of species in the controls (McLaren, Willis, and Callahan (2019)). The measured relative efficiencies can then be used to calibrate (remove the effect of bias from) the relative abundances (ratios) of the control species in a set of primary (non-control) experimental samples that were measured with the same protocol (ideally in the same experiment and sequencing run) (McLaren, Willis, and Callahan (2019)). Calibration can be extended to species not in the controls using statistical methods to impute (predict) the unobserved efficiencies using phylogenetic relatedness, genetic characteristics (such as 16S copy number), and/or phenotypic properties (such as cell-wall structure).
The calibrated compositions can be used for arbitrary downstream microbiome analyses, including relative and absolute DA analysis. To demonstrate the potential for calibration to improve fold-change measurements, we estimated bias from one sample in the mock community data from Brooks et al. (2015) that contained all 7 species and used it to calibrate the compositions of all samples (Figure 5.1). We then estimated species’ densities using the uncalibrated and calibrated proportions using total-abundance normalization, treating the total density as known and fixed. Visual inspection shows that the bias estimated from just a single control community with all species can be sufficient to greatly reduce the effect of taxonomic bias on the measured fold change in species’ proportions and densities (Figure 5.1). To demonstrate a practical application, we used the bias estimated from the full set of mock communities as the basis for calibrating the vaginal community time series measurements from the MOMS-PI study (Fettweis et al. (2019)) that were made using the same 16S sequencing protocol. We imputed the efficiencies of other species using taxonomic relationships (Methods). Calibration then allowed us to explore the impact of bias on the measured trajectories of species proportions within women over the course of pregnancy as described in Section 4.
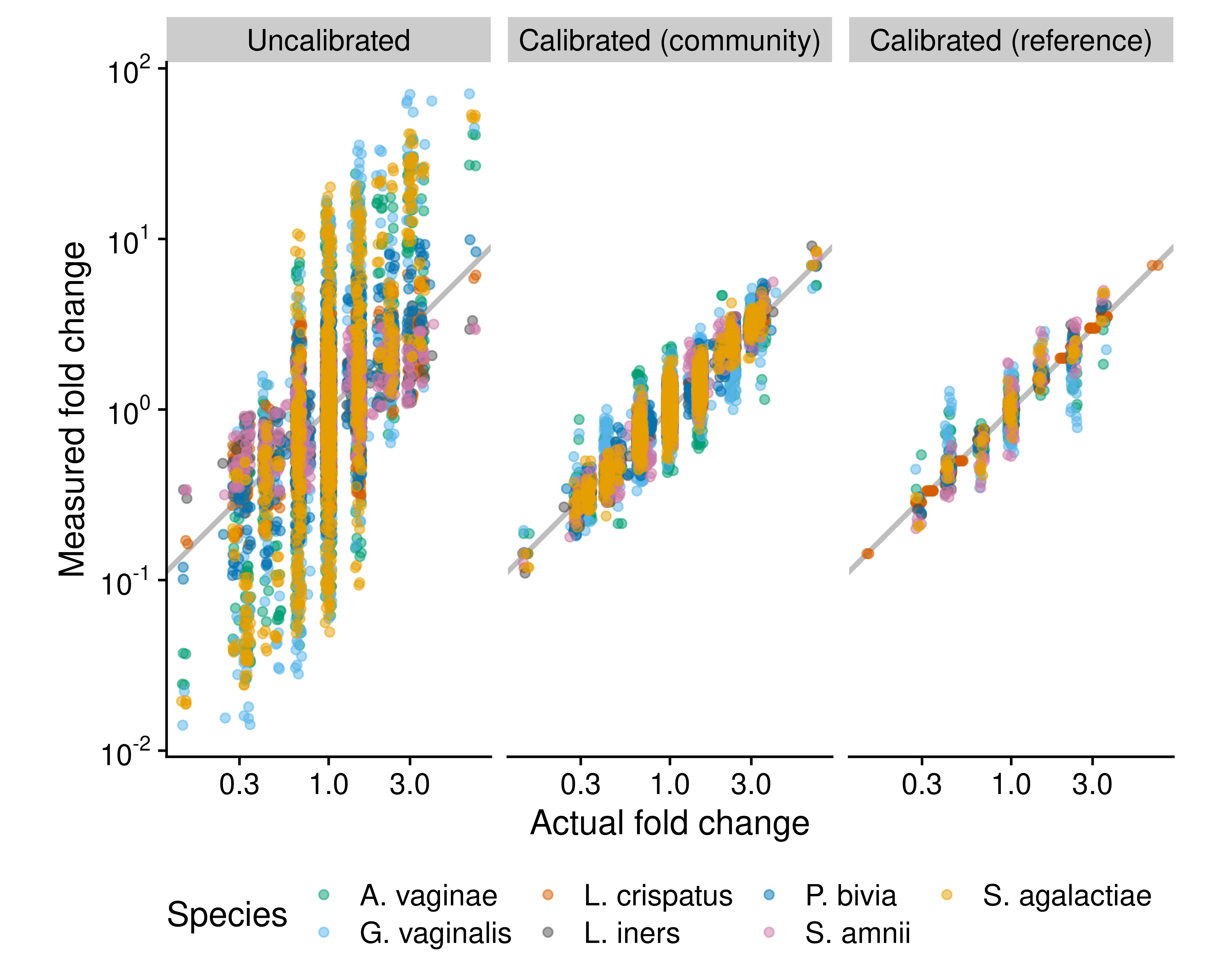
Figure 5.1: Fold changes can be calibrated using community controls or reference species. The figure compares the performance of three methods for measuring fold changes in absolute cell density in cellular mock communities of 7 vaginal species, which were constructed and measured via 16S sequencing by Brooks et al. (2015). The ‘Uncalibrated’ fold changes are derived directly from uncalibrated individual abundance measurements, which equal the product of the species’ proportion by the total density (which here is be known to be constant by construction). The ‘Calibrated (community)’ measurements are computed from abundance measurements where the proportions are first corrected for the taxonomic bias that was estimated from a single sample that contained all 7 species. The ‘Calibrated (reference)’ measurements are computed from abundances measured with the reference-species method, with Lactobacillus crispatus used as the reference; that is, the true abundance of L. crispatus is treated as known and used to infer the abundance of the remaining 6 species. Only samples that contain L. crispatus are included.
5.3 Calibration using reference species
Obtaining control communities that span the taxa of interest is often not practical. Moreover, the bias that is measured from controls may differ from that in the primary samples due to differences in cell state or sample preparation. These problems may be overcome for the purpose of species-level absolute DA analysis by using the reference-species approach to absolute-density measurement that was described in Section 3. This reference-species calibration allows one to simultaneously account for changes in total density and the sample mean efficiency, obtaining accurate fold changes in absolute density from taxonomically biased, relative metagenomics measurements.
Recall that densities estimated by the reference-species approach have a constant fold error, provided that the fold error in the reference species is also constant (Equation (2.14)). Three categories of reference species are reviewed for this purpose in Appendix B, including why they might be expected to produce constant fold errors: housekeeping species assumed to have constant abundance, spike-in species added at known or constant abundance, and species whose abundance has been measured with a targeted method like species-specific qPCR.
To demonstrate the ability for reference calibration to improve fold changes, we treated the abundance of one species (Lactobacillus crispatus) in the Brooks et al. (2015) mock community data as known, and used it to calibrate the abundances of all species. Doing so improved the resulting measurements of fold changes in density for all species (Figure 5.1).
5.4 Choosing complementary total-density and metagenomics measurements
A variety of methods for measuring total community density have been paired with either amplicon or shotgun sequencing data for the purpose of conducting absolute DA analysis using the total-density approach described in Section 3. Each of these methods is subject to its own taxonomic bias: Cells from different species are likely to contribute more or less efficiently to the total abundance measurement. Consider the popular method of assessing bacterial abundance with total-16S qPCR measurement. Even an ideal qPCR protocol that perfectly amplifies all species remains a biased measurement of cell density, since the total 16S copies in the extracted DNA contributed by each species will be proportional to its (species-specific) lysis efficiency and 16S copy-number. 16S qPCR is commonly paired with 16S amplicon sequencing, with which these sources of bias are shared, perhaps along with variation in primer binding and amplification efficiency. Methods like using flow cytometry that directly measures cell density lacks these biases but likely have their own that are more likely to be orthogonal to the 16S sequencing measurement. By extending the analysis of Section 3 to include consistent taxonomic bias in the total-density measurement, we find that pairings of total-density and sequencing measurements that share large sources of taxonomic bias can lead to an offsetting of errors that reduces the error in fold-change measurement and DA inference (Appendix A.2). This finding suggests that methods of total-density measurement that are more accurate as measures of total cell density may actually perform worse than less accurate methods for the purposes of absolute DA inference.
We illustrate with a hypothetical example of two vaginal communities of equal density, but which are dominated either by Lactobacillus iners or Gardnerella vaginalis. Based on our earlier analysis in McLaren, Willis, and Callahan (2019), we estimate that L. iners yields 10X more 16S copies per cell than G. vaginalis in extracted DNA. Ignoring other sources of bias, we expect relative efficiencies of L. iners:G. vaginalis of 10 for both qPCR and 16S sequencing measurements. Consequently, the G. vaginalis community will yield a substantially lower qPCR measurement than the L. iners community and we would incorrectly infer a decrease in total abundance. At the same time, this decrease in the mean efficiency of the sequencing measurement would cause artificially high fold changes in the proportions of all species (Section 3). When using qPCR and 16S sequencing measurements for community-based density estimation, these two opposing errors cancel, yielding accurate fold changes in species densities.
More generally, changes in the mean efficiency of the total-density measurement can offset those in the sequencing measurement when comparing log density of species across samples. If the (log) efficiencies of the total and sequencing measurement are positively correlated across species, then the (log) mean efficiencies will tend to be positively correlated across samples, leading to a reduction in error in DA analysis relative to total-density methods whose efficiencies are uncorrelated with those of the sequencing measurement. These observations suggest that qPCR of a marker gene may be the ideal pairing for amplicon sequencing measurements, despite being a poor measure of changes in total cell density. Similarly, bulk DNA quantification may be an ideal pairing for shotgun sequencing, making it possible to account for variation in lysis efficiency and genome length. These pairings even make it possible to account for error caused by variation among samples in the fraction of unclassified reads, which can form a major fraction of amplicon and shotgun data. Importantly, maximizing the offsetting of errors requires thoughtful choices during bioinformatic analysis, perhaps eschewing the filtering and normalization steps used in many software packages and workflows (Appendix A.2).
5.5 Bias-sensitivity analysis
For experiments that have been conducted without calibration controls, it is still possible to investigate the likelihood that DA results can be explained by taxonomic bias by performing a computational bias-sensitivity analysis.
A bias-sensitivity analysis examines how sensitive the results of a given DA analysis are to the working assumption about the bias of a given protocol. This working assumption is typically is that there is no bias, but could also be bias measured in a previous experiment or predicted from taxonomic features like 16S copy number. A straightforward approach to bias-sensitivity analysis is to use computer simulation to re-analyze the MGS data across a set of simulated taxonomic biases. First, multiple sets of efficiency vectors are randomly generated, each representing a possible quantification of taxonomic bias for the study. Second, each efficiency vector is used to calibrate the original MGS measurements; each set of calibrated measurements represents the true community compositions under the given hypothesized bias. Finally, the DA analysis is re-run on each set of calibrated measurements and the distribution of results is compared graphically or with summary statistics. A graphical summary of such an analysis being applied to results from the corncob frequentist DA- analysis tool ‘corncob’ are shown in SI Figure D.7. The simulated efficiency vectors may be generated to different hypotheses about bias in the given system, such as its overall magnitude and correlation among phylogenetically-related species. Thus the utility of such simulations will increase the more we learn about the properties of taxonomic bias in different systems.
An alternative approach to bias-sensitivity analysis would be to directly include the (unknown) taxonomic bias of the protocol in the statistical model used for DA analysis, as described for bias-aware meta-analysis below. Further development of tools and workflows for performing bias sensitivity analyses could be a valuable way to assay and improve the reliability of microbiome results—for differential abundance and microbiome analyses more generally.
5.6 Bias-aware meta-analysis
So far we have considered analysis of samples subject to the same taxonomic bias; however, meta-analysis of microbiome samples measured across multiple studies must often contend with a diversity of protocols and hence taxonomic biases in samples from different studies. This inconsistency in taxonomic bias across studies poses a problem even for ratio-based analyses. A potential solution is to perform a bias-aware meta-analysis that that explicitly accounts for the possibility of distinct taxonomic bias across studies.
Parametric microbiome meta-analysis models include sets of species-specific latent parameters to model “batch effects”—amorphous differences in the measurements of each study. By configuring these models so that these latent parameters correspond to study-specific species efficiencies, we can improve their biological interpretability and gain the ability to directly assess whether disagreements in DA results across studies can be explained by differences in taxonomic bias. To the extent that taxonomic bias truly is consistent within studies, meta-analyses that directly account for it can have greater statistical power to extract the truly consistent and inconsistent microbial patterns across studies.